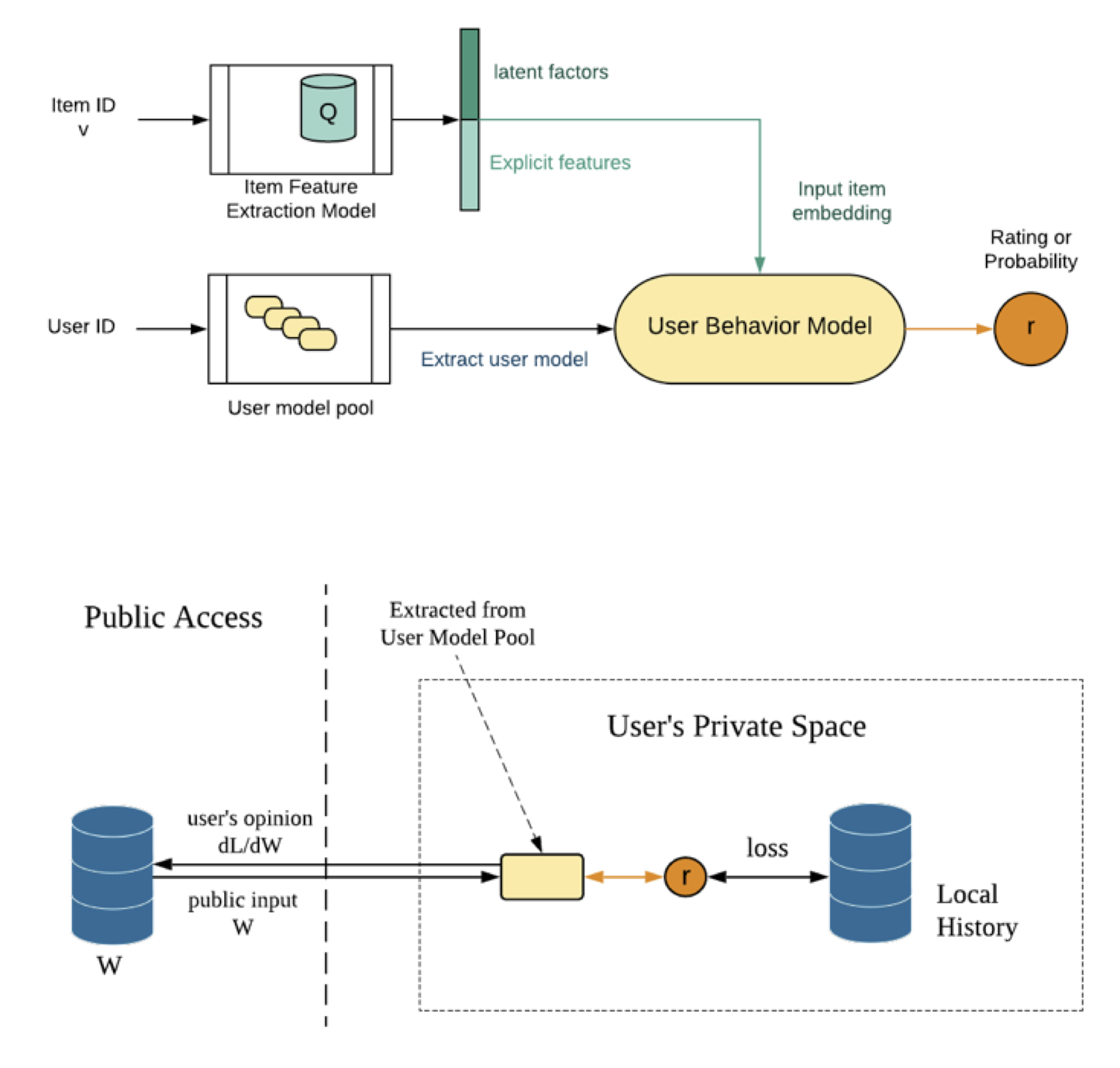
Decentralized Collaborative Filtering
Traditional recommendation and personalization services require service providers to keep a profile of each user, from which they derive individual preferences, as well as aggregated information on multiple users to use for learning common patterns via various collaborative filtering and learning techniques. The recording of users’ private data to built such profiles is the source of increasing concerns from the public in light of numerous data breaches, unethical uses of personal data, and the widespread use of black-box Machine Learning algorithms for decision-making.
Our research focuses on designing privacy-aware user models for collaborative filtering. We aim at designing techniques that allow for the creation of personal user profiles using both information shared with the providers, and private information, kept on the user devices, or personal clouds. We allow users to control which information they are willing to share, and what level of sharing they are comfortable with. Our work centers around Decentralized Collaborative Filtering (DCF) systems that allow for personal recommendations, via collaborative filtering, while leaving sensitive personal data on the users’ own devices.
Project Members
- Amélie Marian
- Yongfeng Zhang
- Shuchang Liu - PhD Student
- Shuyuan Xu - MS Student